Regulatory Reporting
GenAI could be reg reportings’ short-term memory
• 0 minute read
May 5, 2025
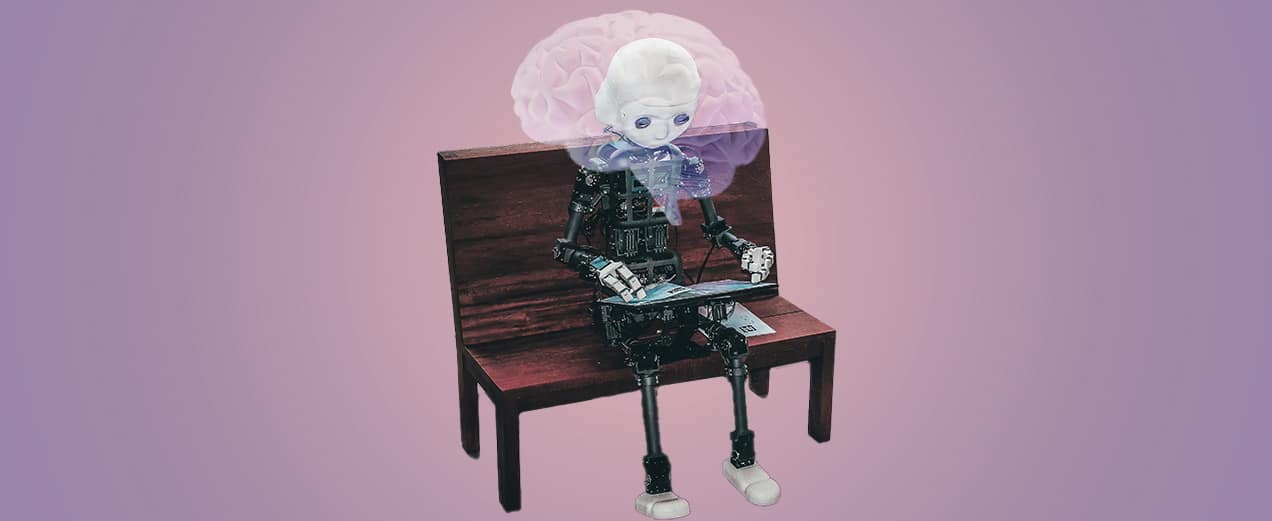
Tools using generative artificial intelligence (GenAI) could become the “short-term memory” for stressed out trade and transaction reporting teams. GenAI tools would act as assistants to trade and transaction subject matter experts (SMEs) and improve information navigation and knowledge retention. That way when SMEs leave a firm institutional knowledge does not leave with them.
Newer large language models (LLMs) can mimic human thought processes, show workings, understand logic and provide attribution. These models are better suited for creating tools to help understand complex regulation and compliance tasks such as trade and transaction reporting, said Pierre Khemdoudi, chief executive at Gentek.AI, a technology company in London developing agentic workflow automation tools.
“Currently customers do knowledge management through SharePoint, possibly with a copilot layer on top,” Khemdoudi said. “But what also happens is people have their own information, their own documents that they use as SMEs. Information is not as standardised as you think.”
Gentek.AI is seeking to provide curated datasets that the GenAI agent can “understand”. GenAI can go through thousands and thousands of pages of regulatory text and help navigate them, but to be reliable and useful for regulatory SMEs, such tools need to be deterministic. This means they cannot ‘hallucinateʼ — generate false or misleading information — and must have the ability, when queried, to provide consistent and correct answers.
Curated datasets related to regulations with trade and transaction reporting obligations, such as the Markets in Financial Instruments Directive (MiFID II), the European Market Infrastructure Regulation (EMIR), the Commodity Futures Trading Commission (CFTC) swaps reporting rules and other similar regimes, can train an LLM to be a specialist in a specific area of regulation or compliance.
“When we looked at building a reg agent, one of the challenges is that the agent needs to understand the difference between types of information such as [regulatory technical standards (RTS)], consultation papers, and other information written by consultants, for example. When new information comes in — RTS, level 1 and 2 texts, best practice — [it] needs to be stored and managed correctly,” Khemdoudi said.
Short-term memory
Reporting SMEs are scarce, and when someone leaves, there is panic because their knowledge leaves with them. The LLM is your short-term memory and brain power: it can retrieve MiFID II and EMIR “memories” in the most efficient and accurate way, according to Khemdoudi.
“Agents will make a huge difference. Agentic tools should be able to relieve some of the stress. It is easier said than done, but I have no doubt this will automate the high stakes, complex compliance tasks. I feel for compliance teams. There is constant activity, constant change and crisis management. There is fatigue. People burn out,” he added.
Three phases
GenAI is not a silver bullet for reporting tasks. It is very good for pre-reporting activity (phase one: analysing, defining and implementing) and post-reporting validation (phase three: quality assurance), but not practical for reporting (phase two) itself, Khemdoudi said.
Post-reporting validation and quality assurance is a MiFID II requirement. Some consultants and technology providers, such as Kaizen in London, already use machine learning to navigate large datasets to find inaccuracies. However, Kaizen is taking a cautious approach to applying AI to its solutions, because of concerns about data privacy and accuracy, said chief product officer Rory McLaren.
The firm has experimented with synthetic transaction reporting data in a secure environment, building an AI tool to search it — but when the Kaizen team compared results with its current tools, the AI tool did not outperform.
“In some areas it was slightly better. [In] some areas it was slightly worse than the existing search capability we had, but when we looked at the cost metrics of how people normally used it, the AI tool would have been 10 times the price of the standard search. And we learned something valuable there, but we didnʼt go ahead and incorporate AI for that, because there was really no viability or utility in it. It was just using AI for AI’s sake,” McLaren said.
Benefits
Speeding up workflows when creating or improving software tools is where McLaren sees the benefits of AI.
“If you break down an end-to-end problem, and you say, Iʼm going to use the AI to make some suggestions at the start and then Iʼm going to pursue them as the expert, or, Iʼm going to come up with my ideas and then Iʼm going to use an AI that Iʼve trained and Iʼve given examples [to], and Iʼm going to ask it to validate what Iʼve done, or check if thereʼs anything that Iʼve missed,” were among the examples he gave.
“But trying to get an AI to [the point where you can simply say] ‘hereʼs some data. Is it valid?’ Thatʼs a pretty heavy lift,” he added. “If you decide how to break the problem up and use it, I think you can definitely get advantages there.”
The UK’s Financial Conduct Authority (FCA) recently launched a AI testing service. The testing service will assess whether firms’ AI tools are ready, helping to accelerate the adoption of AI among financial services providers. It will be launched in September and is expected to run for 12 to 18 months.